Labeling Training Data: The Key to Efficient Data Annotation
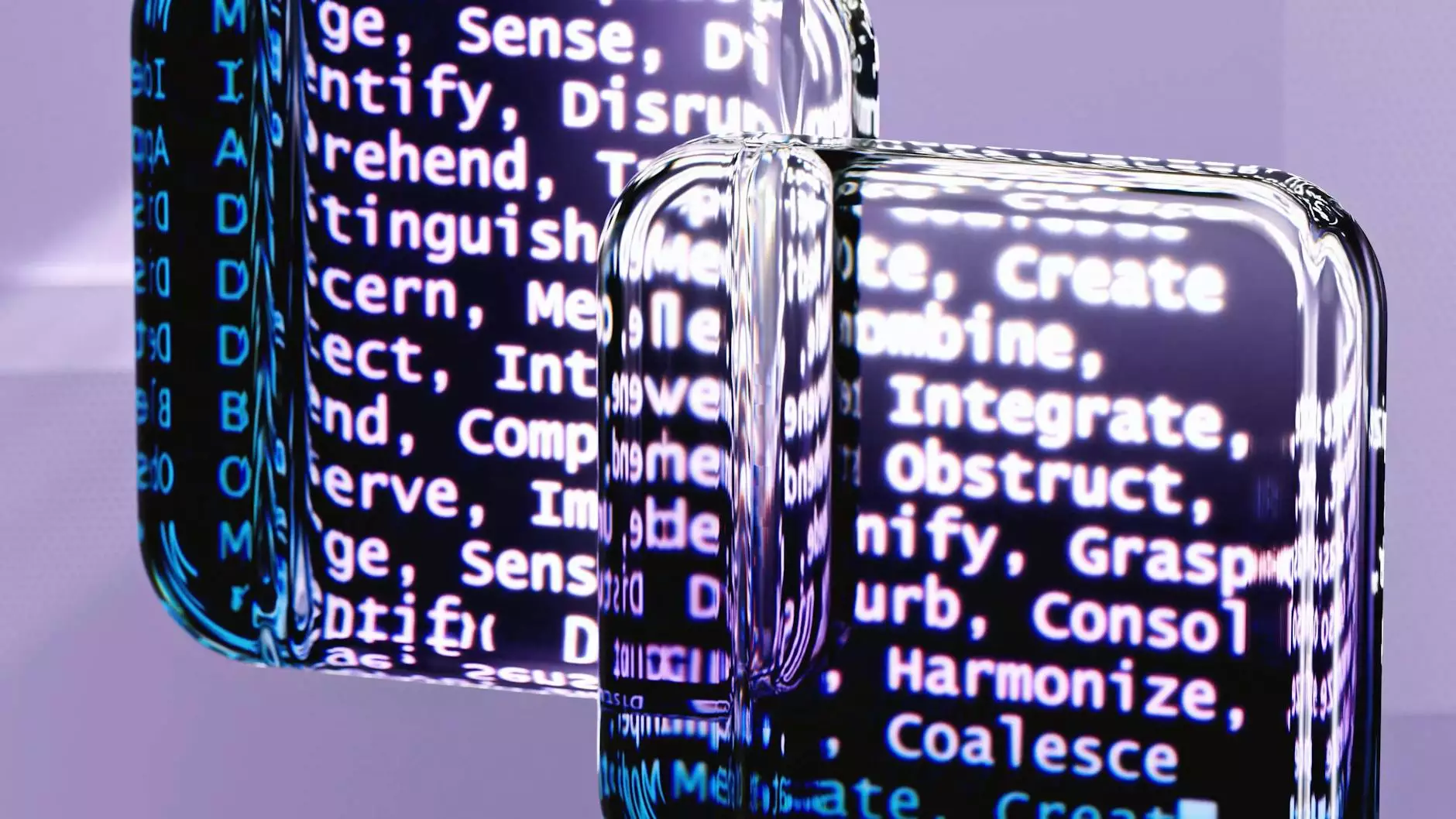
In the rapidly evolving field of artificial intelligence (AI) and machine learning (ML), the phrase labeling training data has emerged as a cornerstone of development. Whether you’re designing an image recognition system, building a natural language processing model, or creating predictive analytics tools, the quality of training data can profoundly impact the performance of your algorithms. This article delves deep into the nuances of labeling training data, why it matters, and how platforms like Key Labs can elevate your data annotation processes.
Understanding the Importance of Training Data
The backbone of any AI system is its training data. This data informs algorithms, enabling them to make decisions and predictions. The significance of labeling training data lies in the fact that it defines the parameters of the learning process. Without accurately labeled data, the AI could misinterpret inputs, leading to flawed outcomes and results that are less reliable.
The Process of Labeling Training Data
Labeling training data involves a systematic approach to tagging datasets with relevant information that guides machine learning models. This process is crucial for supervised learning models, where algorithms learn from labeled examples. Here are some key stages in the labeling process:
- Data Collection: Gather raw data from various sources, which could include images, text, audio, or video.
- Data Preprocessing: Clean and prepare data to eliminate noise and inconsistencies. This may involve removing duplicates, correcting errors, and formatting data uniformly.
- Annotation: Assign labels to the data. This step might be performed manually by human annotators or through automated processes using AI tools.
- Quality Assurance: Review labeled data for accuracy and consistency. This is critical to ensuring that the AI models receive high-quality inputs.
- Dataset Splitting: Divide the annotated data into training, validation, and test sets to ensure effective evaluation of model performance.
Different Types of Data Annotation
The type of data annotation required depends on the specific application of the AI model. Here are several common types of data annotation:
- Image Annotation: Involves tagging images with bounding boxes, polygons, or segmentation masks to help AI understand visual content.
- Text Annotation: This involves labeling text entries for sentiment analysis, named entity recognition, or classification tasks.
- Audio Annotation: Includes transcribing spoken language or identifying sound events within audio files.
- Video Annotation: Involves labeling frames in video feeds, which is essential for applications like surveillance and autonomous vehicles.
The Role of Data Annotation Tools
Investing in the right data annotation tools can significantly enhance the labeling process. Key Labs offers advanced solutions designed to streamline the annotation workflow. Here’s how Key Labs’ data annotation platform stands out:
- Scalability: Easily scale your annotation efforts based on project requirements without compromising quality.
- Collaboration: Facilitate teamwork among annotators, project managers, and data scientists through integrated communication tools.
- Automation: Leverage AI-assisted tools to reduce the manual effort required for labeling, increasing efficiency.
- Customization: Tailor workflows to suit specific project needs, ensuring that unique requirements are met effortlessly.
- Analytics: Access detailed analytics on annotation progress and quality, enabling data-driven decisions and improvements.
Challenges in Labeling Training Data
While labeling training data is essential, it comes with its own set of challenges. Understanding these obstacles can help businesses prepare and adapt their strategies:
1. Time-Consuming Processes
Manually annotating large datasets can be profoundly labor-intensive, leading to delays in project timelines. Businesses often seek efficient workflows that minimize human effort while maximizing output.
2. Ensuring Quality
The accuracy of labels is paramount. Inconsistent or erroneous labeling can mislead AI models, resulting in poor performance. Implementing a robust quality assurance process is essential to mitigate this risk.
3. Domain Expertise
Certain projects require specialized knowledge to label data accurately. For example, medical imaging data might need annotations from healthcare professionals. This requirement can complicate the hiring process for annotators.
4. Managing Large Datasets
As the volume of data increases, so do the complexities involved in managing and annotating it. Leveraging data annotation platforms can help streamline this process and provide better project management capabilities.
Why Choose Key Labs for Labeling Training Data?
When it comes to labeling training data, not all platforms are created equally. Key Labs provides a unique set of advantages that can empower your AI initiatives:
1. Expertise in Annotation
Key Labs has a seasoned team of annotators with diverse expertise across various fields, ensuring that your training data is labeled accurately in accordance with industry standards.
2. State-of-the-Art Technology
With cutting-edge annotation tools, Key Labs can fast-track the data labeling process while maintaining high standards of quality. These tools are designed to assist annotators and improve workflow efficiency.
3. Proven Track Record
Key Labs has a history of successfully delivering annotated datasets for clients across multiple sectors, including healthcare, finance, automotive, and more. This broad experience fosters confidence in their capabilities.
4. Focus on Collaboration
Key Labs promotes a collaborative environment where clients can engage directly with their annotators. This interaction facilitates better understanding and ensures alignment on project goals.
Conclusion: Unlocking Potential
In conclusion, labeling training data is not just a critical step in the machine learning pipeline—it is a catalyst for newfound potential across industries. As AI becomes increasingly prevalent, the necessity for accurately labeled datasets will continue to grow. Leveraging advanced tools and platforms such as Key Labs can streamline this essential process, driving innovation and efficiency in AI and machine learning applications.
In today’s competitive landscape, investing in quality data annotation can distinguish organizations that wish to lead from those that will struggle to keep pace. Don’t leave your AI model’s success to chance; ensure your datasets are well-labeled, reliable, and ready to power the technologies of tomorrow.